Homanga Bharadhwaj
hbharadh at cs dot cmu dot edu
I am a PhD student in the Robotics Institute, School of Computer Science, Carnegie Mellon University advised by Abhinav Gupta and Shubham Tulsiani, and a visiting researcher at Meta AI in the Embodied AI team. I am engaged in the
quest for understanding intelligence by trying to simulate it. Although this quest has kept me fully occupied for the past three years, I also paint, and have a Bachelor of Arts degree in Fine Arts. Some of my paintings can be found here.
I am fortunate to have been advised by fantastic researchers
over the course of my studies. In reverse chronological order, I have been a student researcher at
Google Brain, a research intern at Nvidia Research , a graduate student in the CS Department, University of Toronto and a student researcher at Vector Institute, a research intern at Preferred Networks Inc. Tokyo and an undergrad in the CSE Department, IIT Kanpur. While being an undergrad, I spent time in Mila, Montreal and the National University of Singapore during semester breaks.
Email  / 
Github  / 
GScholar  / 
Twitter  / 
Name  / 
Art  / 
Poem
|
refresh page to see different homangas in different habitats
|
Education / Employment
- [2022 - Present] Visiting Researcher, Meta AI (Pittsburgh)
- [2021 - Present] PhD in Computer Science and Robotics, Carnegie Mellon University (Pittsburgh)
- [Summer, 2021] Student Researcher, Google Brain (remote, Toronto / Mountain View)
- [Spring, 2021] Research Intern, Nvidia Research (remote, Toronto / Santa Clara)
- [2019 - 2021 ] Msc in Computer Science, University of Toronto / Vector Institute (Toronto)
- [Summer, 2019] Research Intern, Preferred Networks, Inc. (Tokyo)
- [Summer, 2018] Research Intern, Mila (Montreal)
- [Winter, 2017] Research Intern, National University of Singapore (Singapore)
- [2015 - 2019 ] BTech in Computer Science and Engineering, IIT Kanpur (Kanpur)
News/Highlights
- [2024] Best Paper in Robot Manipulation Finalist at ICRA 2024
- [2023] Outstanding Presentation Award at the Robot Learning Workshop, NeurIPS 2023
- [2023] Our sample-efficient universal manipulation research was covered by TechCrunch, ACM, IEEE
- [2021] Our research on safe exploration for robotics was convered by VentureBeat
|
If you have any questions / want to collaborate, feel free to send me an email! I am always excited to learn more by talking with people.
|
Research
I'm interested in developing capable robot manipulation systems that learn from raw sensory inputs efficiently, are compliant with human preferences, and can generalize to diverse unseen scenarios. To this end, I have worked on learning goal-conditioned manipulation from human videos, exploration for robot learning, and improving efficiency through semantic augmentations and novel policy architectures. I have eclectic research interests, and have also worked on robustness in machine learning, and improving sample efficiency, scalability, and representations in reinforcement learning.
My research has received a Best Paper in Robot Manipulation Finalist at ICRA, the Outstanding Presentation award at NeurIPS Robot Learning Workshop, and has been covered by several media outlets like TechCrunch, ACM, IEEE, and VentureBeat among others.
|
Robotics / Reinforcement Learning
|
|
RoboAgent: Towards Sample Efficient Robot Manipulation with Semantic Augmentations and Action Chunking
Homanga Bharadhwaj*, Jay Vakil*, Mohit Sharma*, Abhinav Gupta, Shubham Tulsiani, Vikash Kumar
ICRA 2024, Award at NeurIPS 2023 Robot Learning Workshop paper website data  
We can develop a single robot manipulation agent capable of 12 non trivial skills across 100s of scenes, through semantic augmentations for multiplying data, and action chunking transformers for fitting the multi-modal data distribution. We show generalization at different levels of variations including completely new scenarios with an order of magnitude less data compared to prior works.
|
|
Towards Generalizable Zero-Shot Manipulation via Translating Human Interaction Plans
Homanga Bharadhwaj, Abhinav Gupta*, Vikash Kumar*, Shubham Tulsiani*
ICRA 2024 paper website video  
Learning interaction plans from diverse passive human videos on the web, followed by translation to robotic embodiments can help develop a single goal-conditioned policy that scales to over 100 diverse tasks in unseen scenarios, including real kitchens and offices.
|
|
Zero-Shot Robot Manipulation from Passive Human Videos
Homanga Bharadhwaj, Abhinav Gupta, Shubham Tulsiani*, Vikash Kumar*
Spotlight Talk at PTR and RAP4 Workshops, ICRA 2023 paper website  
Learning to predict plausible hand motion trajectories from passive human videos on the web, followed by transformation of the predictions to a robot's frame of reference enables zero-shot coarse-manipulation with real-world objects.
|
|
CACTI: A Framework for Scalable Multi-Task Multi-Scene Visual Imitation Learning
Mandi Zhao, Homanga Bharadhwaj, Vincent Moens, Shuran Song, Aravind Rajeswaran, Vikash Kumar
Pretraining for Robotics Workshop, CoRL 2022 paper website  
Through effective augmentations enabled by recent advances in generative modeling, we can develop a framework for learning robust manipulation policies capable of solving multiple tasks in diverse real-world scenes.
|
|
Visual Affordance Prediction for Guiding Robot Exploration
Homanga Bharadhwaj, Abhinav Gupta, Shubham Tulsiani
ICRA 2023 paper website  
We can enable goal-directed robot exploration in the real world by learning an affordance model to predict plausible future frames given an initial image from passive human interaction videos, in combination with self-behavior cloning for policy learning.
|
|
Simplifying Model-based RL: Learning Representations, Latent-space Models and Policies with One Objective
Raj Ghugare, Homanga Bharadhwaj, Benjamin Eysenbach, Sergey Levine, Ruslan Salakhutdinov
ICLR 2023 paper website reviews  
We can learn a latent-space model, and a policy for RL jointly through a single objective, by deriving a lower-bound to the overall RL objective
|
|
Auditing Robot Learning for Safety and Compliance during Deployment
Homanga Bharadhwaj
CoRL 2022 (Blue Sky Position Paper) paper reviews  
Auditing robot learning algorithms for safety and compliance is important to ensure human compatibility. This position paper argues for increased synergy between the robot learning and AI Alignment communities and describes a preliminary audit framework based on human-in-the-loop learning.
|
|
Information Prioritization Through Empowerment in Visual Model-based RL
Homanga Bharadhwaj, Mohammad Babaeizadeh,Dumitru Erhan, Sergey Levine
ICLR 2022 paper website reviews  
Empowerment along with mutual information maximization helps learn functionally relevant factors in visual model-based RL, especially in environments with complex visual distractors.
|
|
Conservative Safety Critics for Exploration
Homanga Bharadhwaj,
Aviral Kumar, Nicholas Rhinehart,
Sergey Levine, Florian Shkurti,
Animesh Garg
ICLR 2021 paper website reviews  
Training a critic to make conservative safety estimates by over-estimating how unsafe a particular state is, can help significantly minimize the number of catastrophic failures in constrained RL
|
|
Latent Skill Exploration for Planning and Transfer
Kevin (Cheng) Xie*,
Homanga Bharadhwaj*, Danijar Hafner,
Animesh Garg, Florian Shkurti
ICLR 2021 paper website reviews  
Combining online planning of high level skills with an amortized low level policy can improve sample-efficiency of model-based RL for solving complex tasks, and transferring across tasks with similar dynamics.
|
|
Model-Predictive Planning via Cross-Entropy and Gradient-Based Optimization
Homanga Bharadhwaj*,
Kevin (Cheng) Xie*,
Florian Shkurti
L4DC, 2020 paper code reviews  
Updating the top action sequences identified by CEM through a few gradient steps helps improve sample efficiency and performance of planning in Model-based RL
|
|
Continual Model-Based Reinforcement Learning with Hypernetworks
Philip Huang, Kevin (Cheng) Xie, Homanga Bharadhwaj,
Florian Shkurti
ICRA, 2021 and Deep RL Workshop (NeurIPS 20) paper code  
Task-conditioned hypernetworks can be used to continually adapt to varying environment dynamics, with a limited replay buffer in lifelong robot learning
|
|
LEAF: Latent Exploration Along the Frontier
Homanga Bharadhwaj,
Animesh Garg,
Florian Shkurti
ICRA, 2021 paper  
Keeping track of the currently reachable frontier of states, and executing a deterministic policy to reach the frontier followed by a stochastic policy beyond, can help facilitate principled exploration in RL
|
|
D2RL: Deep Dense Architectures in Reinforcement Learning
Samarth Sinha*, Homanga Bharadhwaj*,
Aravind Srinivas,
Animesh Garg
Deep RL Workshop (NeurIPS 20) paper blog code reviews  
Introducing skip connections in the policy and Q function neural networks can improve sample efficiency of reinforcement learning algorithms across different continuous control environments
|
|
MANGA: Method Agnostic Neural-policy Generalization and Adaptation
Homanga Bharadhwaj,
Shoichiro Yamaguchi,
Shin-ichi Maeda
ICRA, 2020 paper  
Training dynamics conditioned policies on dynamics randomized environments and estimating dynamics parameters from off-policy data can help achieve zero-shot adaptation in an unseen test environment
|
|
A Data-Efficient Framework for Training and Sim-to-Real Transfer of Navigation Policies
Homanga Bharadhwaj*,
Zihan Wang*,
Yoshua Bengio,
Liam Paull
ICRA, 2019 paper  
Adversarial domain adaptation can be used for training a gradient descent based planner in simulation and transferrring the learned model to a real navigation environment.
|
Vision / Representation Learning
|
|
DIBS: Diversity inducing Information Bottleneck in Model Ensembles
Samarth Sinha*,
Homanga Bharadhwaj*,
Anirudh Goyal,
Hugo Larochelle,
Animesh Garg,
Florian Shkurti
AAAI, 2021 (and ICML-UDL, 2020) paper  
Explicitly maximizing diversity in ensembles through adversarial learning helps improve generalization, transfer, and uncertainty estimation
|
|
DiVA: Diverse Visual Feature Aggregation for Deep Metric Learning
Timo Milbich*,
Karsten Roth*,
Homanga Bharadhwaj,
Samarth Sinha,
Yoshua Bengio,
Bjorn Ommer,
Joseph Paul Cohen
ECCV, 2020 paper code  
Appropriately augmenting training with multiple complimentary tasks can improve generalization in Deep Metric Learning.
|
|
Generalized Adversarially Learned Inference
Yatin Dandi,
Homanga Bharadhwaj,
Abhishek Kumar,
Piyush Rai,
AAAI, 2021 paper code  
Adversarially learned inference can be generalized to incorporate multiple layers of feedback through reconstructions, self-supervision, and learned knowledge.
|
|
A Generative Framework for Zero Shot Learning with Adversarial Domain Adaptation
Varun Khare,
Divyat Mahajan,
Homanga Bharadhwaj,
VK Verma,
Piyush Rai
WACV, 2020 paper code  
Adversarial Domain Adaptation appropriately incorporated in a Generative Zero Shot Learning model can help minimize domain shift and significantly enhance generalization on the unseen test classes
|
|
Auditing AI models for Verified Deployment under Semantic Specifications
Homanga Bharadhwaj,
De-An Huang,
Chaowei Xiao,
Anima Anandkumar
Animesh Garg
under review paper blog  
Auditing deep-learning models for human-interpretable specifications, prior to deployment is important in preventing unintended consequences. These specifications can be obtained by considering variations in an interpretable latent space of a generative model.
|
|
RecGAN: Recurrent Generative Adversarial Networks for Recommendation Systems
Homanga Bharadhwaj,
Homin Park,
Brian Y. Lim
RecSys, 2018 paper
Recurrent Neural Network based Generative Adversarial Networks can learn to effectively model the latent preference trends of users in time-series recommendation.
|
My tryst with HCI research
|
|
De-anonymization of authors through arXiv submissions during double-blind peer review
Homanga Bharadhwaj,
Dylan Turpin,
Animesh Garg,
Ashton Anderson
arXiv, 2020 paper blog  
In an analysis of ICLR 2020 and 2019 papers, we find positive correlation between releasing preprints on arXiv and acceptance rates of papers by well-known authors. For well known authors, acceptance rates for papers with arxiv preprint are higher than those without preprints released during review.
|
|
New tab page recommendations cause a strong suppression of exploratory web browsing behaviors
Homanga Bharadhwaj,
Nisheeth Srivastava
WebSci, 2019
Passive website recommendations embedded in the new tab displays of browsers (that recommend based on frecency) inhibit peoples' propensity to visit diverse information sources on the internet
|
My freshman year dabble with Quantum Entanglement
|
Codes
|
Teaching Experience and Service
|
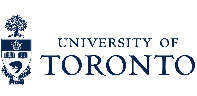 |
Teaching Assitant (TA),
Introduction to Mobile Robotics (CSC477), Fall 2020
Teaching Assitant (TA),
Algorithmic Intelligence in Robotics (CSC375), Fall 2020
|
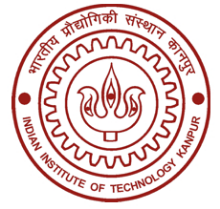 |
Teaching Assitant (TA),
Computational Cognitive Science (CS786), Winter 2019
Course Project Mentor,
Topics in Probabilistic Modeling and Inference (CS698), Winter 2019
Course Project Mentor,
Introduction to Machine Learning (CS771), Autumn 2018
|
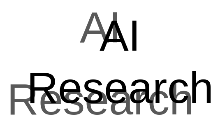 |
Student Volunteer at
ACM RecSys 2018, Vancouver, BC
Student Volunteer at
ACM SIGIR 2018, Ann Arbor, Michigan
Reviewer for Computers in Human Behavior , ICRA 2019 , ICRA 2020, IROS 2020, CoRL 2020, ACM ToCHI
|
I love his website design.
|
|